Release Notes
Discover what's new
in nQuery
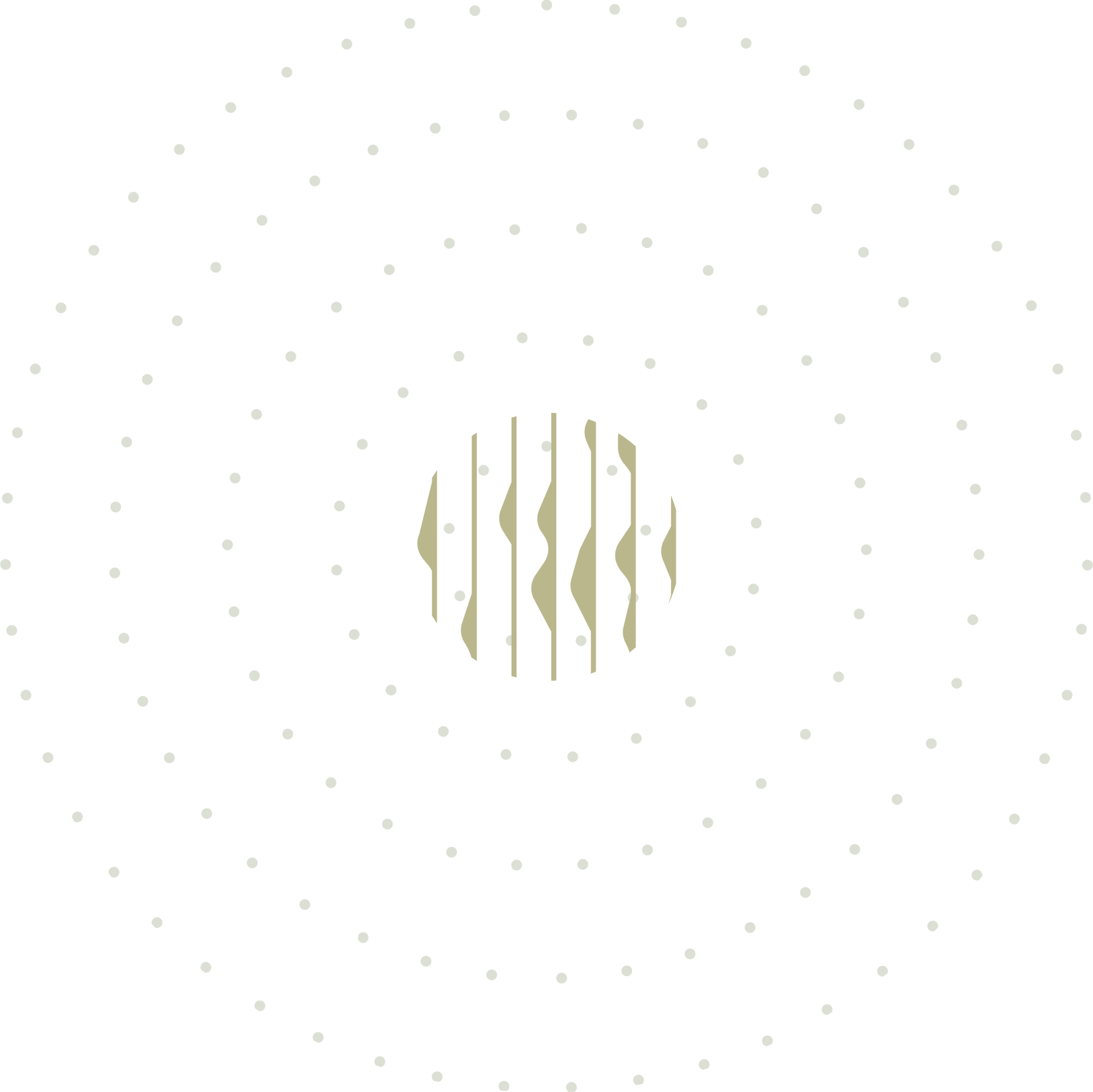
Version 9.4
From fixed to flexible trial designs, nQuery 9.4 is a major update to help biostatisticians and clinical researchers save costs and reduce risk.
Highlights include:
- A simulation tool for sequential design operating characteristics
- An upgraded survival analysis group sequential design table
- Multiple new options for mixed models
Jump to Section
nQuery Pro Tier
What's new in the PRO tier of nQuery?
2 new sample size tables have been added to the Pro tier of nQuery 9.4.
- Group Sequential Trial Design Overhaul for Survival analysis
- Simulation Tool for Sequential Design Operating Characteristics
1. Group Sequential Design Overhaul For Survival Analysis
What is it?
Group Sequential Design is the most common adaptive design used in confirmatory clinical trials. This design allows trialists to stop a trial early at pre-specified interim analyses if there is sufficient evidence that treatment is effective (efficacy) or ineffective (futility). Group sequential designs capacity to stop trials early can lead to significant cost savings while also getting vital treatments into the hands of patients faster. Methods such as the Lan-DeMets error spending function give trialists significant flexibility to define the conditions under which a trial will stop early while maintaining significant flexibility during trial monitoring.
Survival analysis is one of the most common endpoints in clinical trials especially in areas such as oncology. Survival analysis has unique challenges for power and sample size determination both in fixed term and sequential trials in areas such as modelling accrual processes, dealing with dropout and adjusting for different types of censoring.
nQuery 9.4 adds an overhauled group sequential table for the two-sample log-rank test, building upon the tables added for the two means and two proportions scenarios in nQuery 9.3. This overhaul includes a number of additional group sequential methods, substantial improvements in user experience and additional detailed outputs to better explore different group sequential scenarios. The new group sequential survival analysis table deals allows for piecewise accrual, hazard rates and dropout rates and accommodates both event-driven and fixed follow-up designs while providing detail on the expected sample size, events and analysis timing.
Future updates will expand to additional endpoints such as counts and one arm designs.
Group Sequential Trial Design Overhaul Summary:
- Group Sequential Design for Two Sample Log-Rank Test (Updated Table)
- 11 Spending Functions (O’Brien-Fleming, Pocock, Power Family, Hwang-Shih-DeCani, Exponential, Beta, t-distribution, Logistic, Normal, Cauchy, User Defined/Interpolated)
- Wang-Tsiatis & Pampallona-Tsiatis Designs
- Haybittle-Peto (p-value) Design
- Unified Family Design
- Custom Boundary Design with custom Z statistic, p-value, Score Statistic or Effect Size boundary inputs
- 2-sided Futility Boundaries
- New user-responsive interface
- Boundary Parameterization Conversion
- Detailed & Exportable Group Sequential Reports
- Improved Editable Boundary Plots
- Error Spending Plots
2. Simulation Tool for Sequential Design Operating Characteristics
What is it?
Group Sequential Design is the most common adaptive design used in confirmatory clinical trials. This design allows trialists to stop a trial early at pre-specified interim analyses if there is sufficient evidence that treatment is effective (efficacy) or ineffective (futility). Group sequential designs capacity to stop trials early can lead to significant cost savings while also getting vital treatments into the hands of patients faster.
Simulation is considered a key aspect of evaluating the statistical and practical performance of adaptive designs. By using simulation you can flexibly evaluate a design over a wide variety of scenarios and gain valuable insights into the pros and cons of your complex design. nQuery 9.4 adds a tool for evaluating the operating characteristics of group sequential designs such as average power and sample size and the number of times you can expect at a given interim analysis. This tool can be used separately or can automatically take values from your existing group sequential design from the overhauled suite of group sequential tables.
Future updates will expand this simulation tool to additional adaptive designs, group sequential design endpoints and additional simulation options.
Tool Added:
- Simulation Tool for Sequential Design Operating Characteristics
How to update?
If you have a subscription for the Query Pro Tier nQuery should automatically prompt you to update. You can manually update nQuery Advanced by clicking Help>Check for updates.
To discuss any aspect of your subscription, click here.
nQuery Base Tier
What's new in the BASE tier of nQuery?
13 new sample size tables/updates have been added to the Base tier of nQuery 9.4 in the following areas:
- Survival (3 Updates)
- Mixed Models (7 Updates)
- Correlation (2 Updates)
- Confidence Intervals (1 Update)
3. Survival (Time-to-Event) Analysis
What is it?
Survival or Time-to-Event trials are trials in which the endpoint of interest is the time until a particular event occurs, for example death or tumour regression. Survival analysis is often encountered in areas such as oncology or cardiology.
In nQuery 9.4, sample size tables are added in the following areas for the design of trials involving survival analysis. Future updates will expand to additional endpoints such as counts and one arm designs.
Tables/Features added:
- Log-Rank Test with Fixed Follow-up
- Modestly Weighted Linear-Rank Test (MWLRT)
4. Log-Rank Test with Fixed Follow-up
What is it?
The log-rank test is one of the most common statistical tests used for the analysis of survival data. Its flexibility and interpretability provide useful insights into the comparable hazard rates in survival trials.
In nQuery 9.4, two sample size tables are added which build on the existing log-rank options in nQuery. These tables focus on allowing for fixed follow-up designs where subjects are censored after a fixed period of the time. These updated tables allow for either accrual percentage or accrual rate inputs and for piecewise accrual, hazard rate and dropout process with either rounded or unrounded sample size and event outputs.
Table(s) Added:
- Log-Rank Test, User-Specified Accrual %
- Fixed Follow-up, Piecewise Survival and Dropout Rates
- Log-Rank Test, User-Specified Accrual Rates, Fixed Follow-up
- Piecewise Survival and Dropout Rates
5. Modestly Weighted Linear-Rank Test
What is it?
The log-rank test is one of the most widely used tests for the comparison of survival curves. However, a number of alternative linear-rank tests are available. The most common reason to use an alternative test is that the performance of the log-rank test depends on the proportional hazards assumption and may suffer significant power loss if the treatment effect (hazard ratio) is not constant. While the standard log-rank test assigns equal importance to each event, weighted log-rank tests apply a prespecified weight function to each event. However, there are many types of non-proportional hazards (delayed treatment effect, diminishing effect, crossing survival curves) so choosing the most appropriate weighted log-rank test can be difficult if the treatment effect profile is unknown at the design stage.
Proposals to deal with non-proportional hazards include the MaxCombo design, RMST and piecewise weighted models. One proposal made in response to MaxCombo was the modestly weighted linear-rank test (MLWRT) which avoids the lack of strong Type I error seen in MaxCombo while maintaining the strength of flexibility regarding the timing of a delayed effect.
In nQuery 9.4, the MWLRT is added as an additional test option to our suite of MaxCombo and linear-rank test tables which are available for inequality, non-inferiority and equivalence hypotheses.
Feature added to MaxCombo and Linear-Rank tables:
- Modestly Weighted Linear-Rank Test (MLWRT)
6. Mixed Models
What is it?
Mixed models are models used when data is nested within multiple levels such as hierarchical and repeated measures designs. For example, when students (level 1) are nested within a class (level 2) which are nested within a school (level 3) which are nested within a school district (level 4). These are also known as multi-level or mixed-effects models.
For example, a common type of hierarchical trial design would be a 2-Level longitudinal hierarchical design in which subjects (level 2) are randomized to one of two treatments and then multiple observations (level 1) are made on each subject over time. In determining the sample sizes for such a design, the hierarchical data structure must be considered since both the first and second level units contribute to the total variation in the observed outcomes. In addition, level 1 data units (i.e. repeated measurements) from the same level 2 unit 9 (i.e. subject) tend to be positively correlated.
In nQuery 9.4, 7 new Mixed Model tables for End of Follow-up and Factorial 2x2 are added based on Ahn, Heo, and Zhang (2015).
Tables added:
- Mixed Models Test for Two Means at the End of Follow-Up in a 2-Level Hierarchical Design (Level 2 Randomization)
- Mixed Models Test for Two Means at the End of Follow-Up in a 3-Level Hierarchical Design (Level 3 Randomization)
- Mixed Models Test for Interaction Effect in 2x2 Factorial Design in a 3-Level Hierarchical Design (Level 3 Randomization)
- Mixed Models Test for Interaction Effect in 2x2 Factorial Design in a 3-Level Hierarchical Design (Level 2 Randomization)
- Mixed Models Test for Interaction Effect in 2x2 Factorial Design in a 3-Level Hierarchical Design (Level 1 Randomization)
- Mixed Models Test for Interaction Effect in 2x2 Factorial Design in a 2-Level Hierarchical Design (Level 2 Randomization)
- Mixed Models Test for Interaction Effect in 2x2 Factorial Design in a 2-Level Hierarchical Design (Level 1 Randomization)
7. Correlation
What is it?
Correlations measures are interested in assessing the strength of the relationship between two variables. Common correlation measures include Pearson, Spearman and Kendall-Rank.
Dependent correlations indicate a common factor that may lead to a relationship between the two correlations which is equivalent to stating that at least one of the pairwise correlation coefficients is non-zero. When two correlations have a measurement index in common (here designated "y") then by comparison of correlations of two other measurements against the common measurement index must be dependent. In nQuery 9.4, 2 tables are added for tests of correlation between dependent (paired) measurements.
Tables added:
- Test for Two Dependent Pearson Correlations (Common Index)
- Test for Two Dependent Pearson Correlations (No Common Index)
8. Confidence Intervals
What is it?
Confidence Intervals are the most widely used statistical interval and represent an interval within which a population parameter is likely to lie with a given level of probability.
Count and incidence rates are a common type of data where the endpoint of interest is the number of events that occur in a given unit of time or repeated counts. Examples in clinical trials include the exacerbation incidence rate in a given year for respiratory diseases such as COPD or the count of the number of lesions found in MRI scans for conditions such as multiple sclerosis. Count models such as Poisson or Negative Binomial allow the full usage of all events found compared to binomial or survival modelling approaches. In nQuery 9.4, 1 table is added for the confidence interval for single Poisson incidence rate.
Tables added:
- Confidence Interval for One Poisson Rate
How to update?
nQuery should automatically prompt you to update. You can manually update nQuery Advanced by clicking Help>Check for updates.
To discuss any aspect of your subscription, click here.
nQuery Predict
Accurately predict your key trial milestones. Identify roadblocks and take action to keep your trial on schedule.
nQuery Predict is the most recent module to be added to the nQuery platform for clinical trial design.
Only available in the expert tier, nQuery Predict is a suite of tools that uses current data to project the likely trajectory of future enrollment or event milestones. With the nQuery Predict module, you can make more informed decisions based on real trial data as it becomes available.
Listen to the short video below for more information about milestone prediction.
Get started with nQuery today
Start for free and upgrade as your team grows